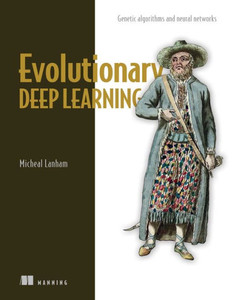
Embedded Deep Learning: Algorithms, Architectures And Circuits For Always-On Neural Network Processing
Springer
ISBN13:
9783030075774
$108.02
This book covers algorithmic and hardware implementation techniques to enable embedded deep learning. The authors describe synergetic design approaches on the application-, algorithmic-, computer architecture-, and circuit-level that will help in achieving the goal of reducing the computational cost of deep learning algorithms. The impact of these techniques is displayed in four silicon prototypes for embedded deep learning. Gives a wide overview of a series of effective solutions for energy-efficient neural networks on battery constrained wearable devices; Discusses the optimization of neural networks for embedded deployment on all levels of the design hierarchy - applications, algorithms, hardware architectures, and circuits - supported by real silicon prototypes; Elaborates on how to design efficient Convolutional Neural Network processors, exploiting parallelism and data-reuse, sparse operations, and low-precision computations; Supports the introduced theory and design concepts by four real silicon prototypes. The physical realization's implementation and achieved performances are discussed elaborately to illustrated and highlight the introduced cross-layer design concepts.
- | Author: Bert Moons, Daniel Bankman, Marian Verhelst
- | Publisher: Springer
- | Publication Date: Jan 19, 2019
- | Number of Pages: 222 pages
- | Language: English
- | Binding: Paperback
- | ISBN-10: 303007577X
- | ISBN-13: 9783030075774
- Author:
- Bert Moons, Daniel Bankman, Marian Verhelst
- Publisher:
- Springer
- Publication Date:
- Jan 19, 2019
- Number of pages:
- 222 pages
- Language:
- English
- Binding:
- Paperback
- ISBN-10:
- 303007577X
- ISBN-13:
- 9783030075774